Generative AI: Use cases, applications, solutions and implementation
Generative AI demonstrates versatile applications across diverse industries, leveraging its capacity to create novel content, simulate human behavior, and generate innovative outputs based on learned patterns.
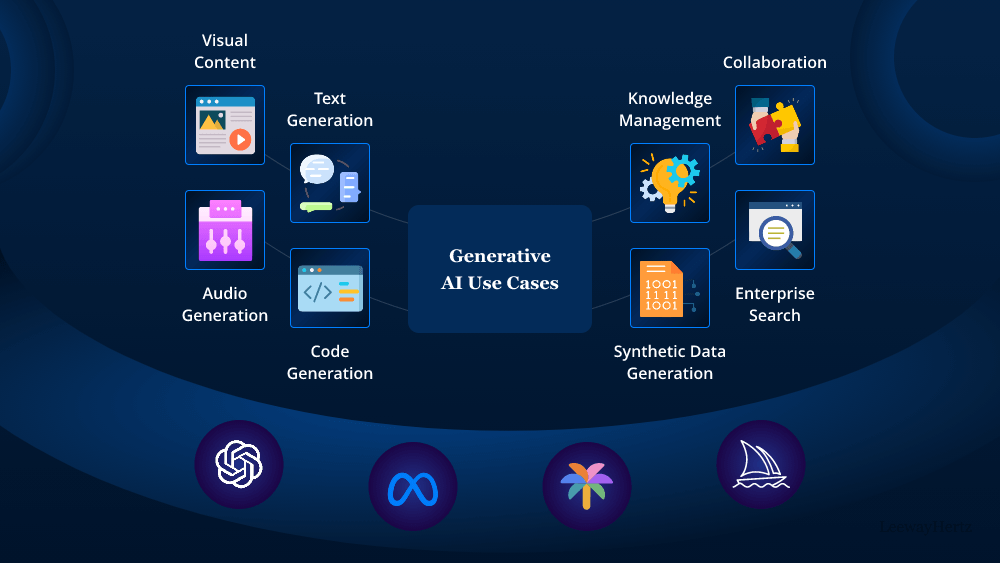
How to train an open-source foundation model into a domain-specific LLM?
A domain-specific language model constitutes a specialized subset of large language models (LLMs), dedicated to producing highly accurate results within a particular domain.
Accelerating AI model training with transfer learning
Transfer learning is a machine learning approach that involves utilizing knowledge acquired from one task to improve performance on a different but related task.
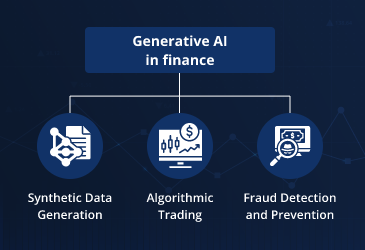
Generative AI in finance and banking: The current state and future implications
The finance industry has embraced generative AI and is extensively harnessing its power as an invaluable tool for its operations.
Demystifying diffusion Models: A comprehensive guide to key concepts and applications
Unlike GANs, diffusion models require only a single model for training and image generation, making them less complex and more efficient for image generation applications.
A comprehensive guide on foundation models
A foundation model is a deep learning algorithm that undergoes pre-training on a massive and diverse dataset, such as images or text.
Prioritizing security in AI development: Training, building, and deploying models in a secure environment
Companies achieve maximum AI model security by integrating robust security protocols, following best practices, and ensuring adherence to regulations.
The current state of Generative AI: A comprehensive overview
The current state of generative AI is filled with exciting possibilities, albeit accompanied by challenges. The industry’s concerted efforts in overcoming these hurdles promise a future where generative AI technology becomes an integral part of our everyday lives.
A comprehensive exploration of various machine learning techniques
A machine learning algorithm is a set of mathematical rules and procedures that allows an AI system to perform specific tasks, such as predicting output or making decisions, by learning from data.
Bayesian networks in AI: Role in machine learning, example, types and applications
Bayesian networks are graphical models utilizing a Directed Acyclic Graph (DAG) to represent a group of random variables and their probabilistic relationships.
What is explainable AI? Use cases, benefits, models, techniques and principles
Explainable AI refers to a collection of processes and techniques that enable humans to comprehend and trust the outputs generated by machine learning algorithms.
How to use LLMs in synthesizing training data?
Harnessing the power of large language models (LLMs), a mighty tool capable of understanding, generating, and even refining human-like text we can generate synthesized training data that is flawless and train our models more efficiently.
Pattern recognition in ML: A comprehensive overview
Pattern recognition in machine learning enables systems to discover similarities and automatically extract meaningful information from a given dataset.